AI’s Role in Pioneering New Enzyme Technologies
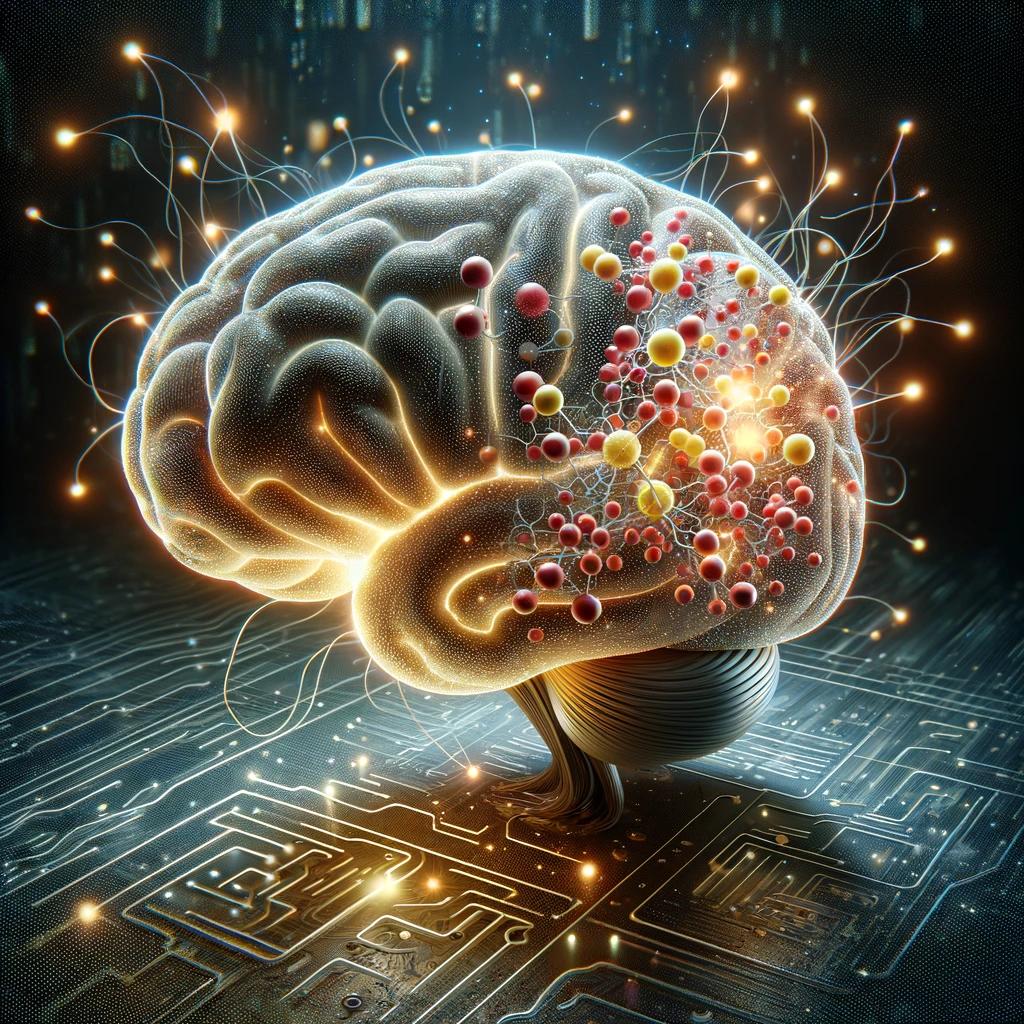
Enzymes are nature’s catalysts, essential for speeding up chemical reactions in biological systems. Understanding and engineering these complex molecules have always been challenging due to their intricate structures and functions. A recent paper titled “Harnessing Generative AI to Decode Enzyme Catalysis and Evolution for Enhanced Engineering“ introduces an innovative approach to enzyme engineering using generative artificial intelligence (AI).
The Power of Generative AI in Enzyme Engineering
Generative AI models excel at learning patterns from vast amounts of data, making them valuable tools in fields like image recognition and natural language processing. In enzyme engineering, generative AI can analyze extensive datasets of enzyme structures and functions to identify patterns that might be overlooked by human researchers.
By leveraging these patterns, AI can predict how changes in an enzyme’s amino acid sequence—known as mutations—will affect its function. This predictive capability allows scientists to virtually assess the impact of specific mutations before conducting laboratory experiments, saving time and resources.
Predicting the Impact of Mutations
Mutations are small changes in the enzyme’s building blocks that can significantly alter its activity. Traditionally, understanding the effects of mutations required extensive experimental work. Generative AI models can simulate these changes and predict their outcomes, acting like a “crystal ball” for enzyme design.
For example, AI can help determine whether swapping one amino acid for another will enhance the enzyme’s catalytic efficiency or stability. This insight guides researchers in creating more effective enzymes for specific applications.
Exploring Enzyme Evolution
The paper also discusses how AI can provide insights into enzyme evolution. By analyzing evolutionary data, AI models can trace how enzymes have adapted over millions of years. This information helps scientists understand the natural mechanisms of enzyme optimization and apply similar principles to engineer new enzymes with desired properties.
Real-World Applications
The integration of AI and enzyme engineering has the potential to revolutionize various industries:
- Medicine: Designing enzymes that can produce new pharmaceuticals or improve drug delivery systems.
- Environmental Protection: Creating enzymes capable of breaking down pollutants or recycling plastics.
- Energy Production: Engineering enzymes that facilitate the production of biofuels and other renewable energy sources.
These applications demonstrate how AI-driven enzyme engineering can address critical global challenges, from healthcare advancements to sustainable environmental solutions.
Future Directions
While significant progress has been made, the paper emphasizes the need for more extensive datasets and advanced AI algorithms. As more enzyme data becomes available and AI technology continues to evolve, the accuracy and capabilities of predictive models will improve.
Future research may focus on:
- Enhancing AI models to predict complex enzyme behaviors.
- Integrating experimental feedback to refine AI predictions.
- Expanding the scope to design entirely new enzymes for novel functions.
Harnessing generative AI for enzyme engineering represents a transformative step in biotechnology. By combining the power of AI with biological science, researchers can design enzymes with unprecedented precision and efficiency. This synergy opens up exciting possibilities for innovation across multiple sectors, potentially leading to breakthroughs that can significantly benefit society.
References
- Original Research Paper: Harnessing Generative AI to Decode Enzyme Catalysis and Evolution for Enhanced Engineering.
Keywords
Enzyme Engineering, Generative AI, Artificial Intelligence, Enzyme Catalysis, Mutation Prediction, Enzyme Evolution, Biotechnology, Synthetic Biology
Disclaimer: The information presented in this article is for educational purposes and reflects current research findings. For detailed information, please refer to the original research paper or consult a professional in the field.