AlphaFold 3: Pioneering the Future of Biological Research
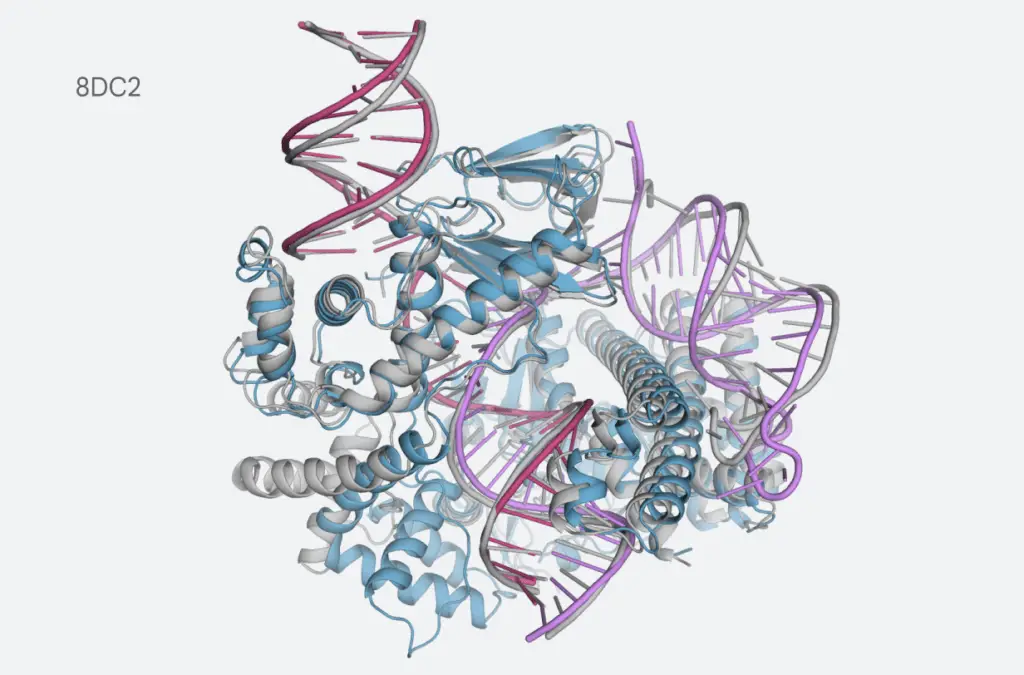
In a recent paper published in Nature, Google DeepMind and Isomorphic Labs unveiled AlphaFold 3, the latest iteration of their groundbreaking AI model for predicting the three-dimensional structures of biomolecular complexes. This advanced model extends beyond proteins to include DNA, RNA, and small molecules, enhancing both the accuracy and scope of molecular interaction predictions. Researchers can explore AlphaFold 3’s capabilities through the newly launched AlphaFold Server, which offers an accessible interface for generating detailed molecular complex predictions.
Enhanced Capabilities and Innovations
AlphaFold 3 represents a substantial advancement in modeling complex biological systems. Unlike its predecessor, AlphaFold 2, which was primarily limited to predicting individual protein structures, AlphaFold 3 can accurately model interactions between various biomolecules. This capability is crucial for understanding cellular mechanisms and developing new drugs. For instance, the model’s enhanced accuracy in predicting protein-ligand and protein–nucleic acid interactions surpasses previous methods.
A key innovation in AlphaFold 3 is its use of a diffusion network. Similar to techniques used in AI image generators, this approach starts with a noisy representation of atoms and refines it step by step to produce accurate molecular structures. This method allows AlphaFold 3 to handle a broader range of inputs and complex interactions more effectively.
Impact on Drug Discovery and Biotechnology
The model’s ability to predict structures with atomic accuracy offers transformative potential in drug discovery and biotechnology. By accurately modeling how drugs bind to their targets, AlphaFold 3 can accelerate the development of new therapeutics for diseases such as cancer and genetic disorders. Early results indicate that AlphaFold 3 outperforms existing docking methods, which are commonly used but are less flexible and accurate.
Challenges and Limitations
Despite these advancements, AlphaFold 3 is not without limitations. The model’s predictions can vary in accuracy, particularly for protein–RNA interactions, and it may occasionally generate non-existent structures—a phenomenon known as “hallucination.” Additionally, Google DeepMind has opted not to release the full code for AlphaFold 3, instead providing access through a controlled server interface. This decision may limit its utility for some researchers who require direct interaction with the software.
AlphaFold 3’s development marks a significant milestone in computational biology, offering unprecedented accuracy and broad applicability in predicting biomolecular structures. Its impact on scientific research and drug discovery is poised to be substantial, potentially accelerating the development of new treatments and deepening our understanding of complex biological systems. While challenges remain, AlphaFold 3 sets a new standard in the field of structural biology.
References
- Google DeepMind
- Isomorphic Labs.
- The server is available at AlphaFold Server
- Original Research Paper
Keywords
AlphaFold 3, Biomolecular Structures, Protein Folding, Computational Biology, Deep Learning, Drug Discovery, Diffusion Network, Molecular Interactions, Structural Biology, Google DeepMind
Disclaimer: The information presented in this article is for informational purposes only and reflects the findings of the referenced study as of the publication date. For detailed information, please refer to the original research paper or consult professionals in the field.